Code of Conduct
As all Brainhacks, BrainHack Marseille is
dedicated to providing a harassment-free Brainhack experience for everyone,
regardless of gender, gender identity and expression, sexual orientation, disability, physical appearance,
body size, race, age or religion.
We do not tolerate harassment of event participants in any form.
Sexual language and imagery is not appropriate for any event venue, including talks.
Event participants violating these rules may be sanctioned or expelled from the event.
Register
Registration is now closed!
Program
- Python for beginners
- Version control using Datalad + BIDS
- ViT (Vision Transformers) - a library combining transformers for image processing
- Edge AI - for an overview of advances in AI and a few demonstrations
- Introduction to Arduino
Wednesday 27th November
09h00-09h45 | Welcome to BrainHack Marseille 2024
Location: Room 507, 5th floor, Bâtiment Pédagogique
|
---|---|
09h45-13h00 | Training session 1
|
13h00-14h00 | Lunch Break (1h00) | 14h00-16h30 | Training Session 2
|
16h30-16h55 | Coffee Break (25 min) |
16h55-18h10 |
Training Session 3
|
18h10-18h30 | Short projects presentations (20 min) |
18h30 | BHM social event with a collective intelligence game!
Location: INT, 5th floor |
Thursday 28th November
09h00-13h00 | Project Work
Location: Room 507, 5th floor, Bâtiment Pédagogique
|
---|---|
13h00-14h00 | Lunch Break |
14h00-18h00 | Project Work
|
18h00-19h00 | Round table Discussion
|
Friday 29th November
09h00-13h00 | Project Work
Location: Room 507, 5th floor, Bâtiment Pédagogique
|
---|---|
13h00-14h00 | Lunch Break | 14h00-18h00 | Project Work
|
Projects
Here you can find all the informations about the event projects.
If you want to submit a project you should follow the link, fill the form, and open a github issue.
Projects can be anything you'd like to work on during the event with other people (coding, discussing a
procedure with coworkers, brainstorming about a new idea), as long as you're ready to minimally organize
this!
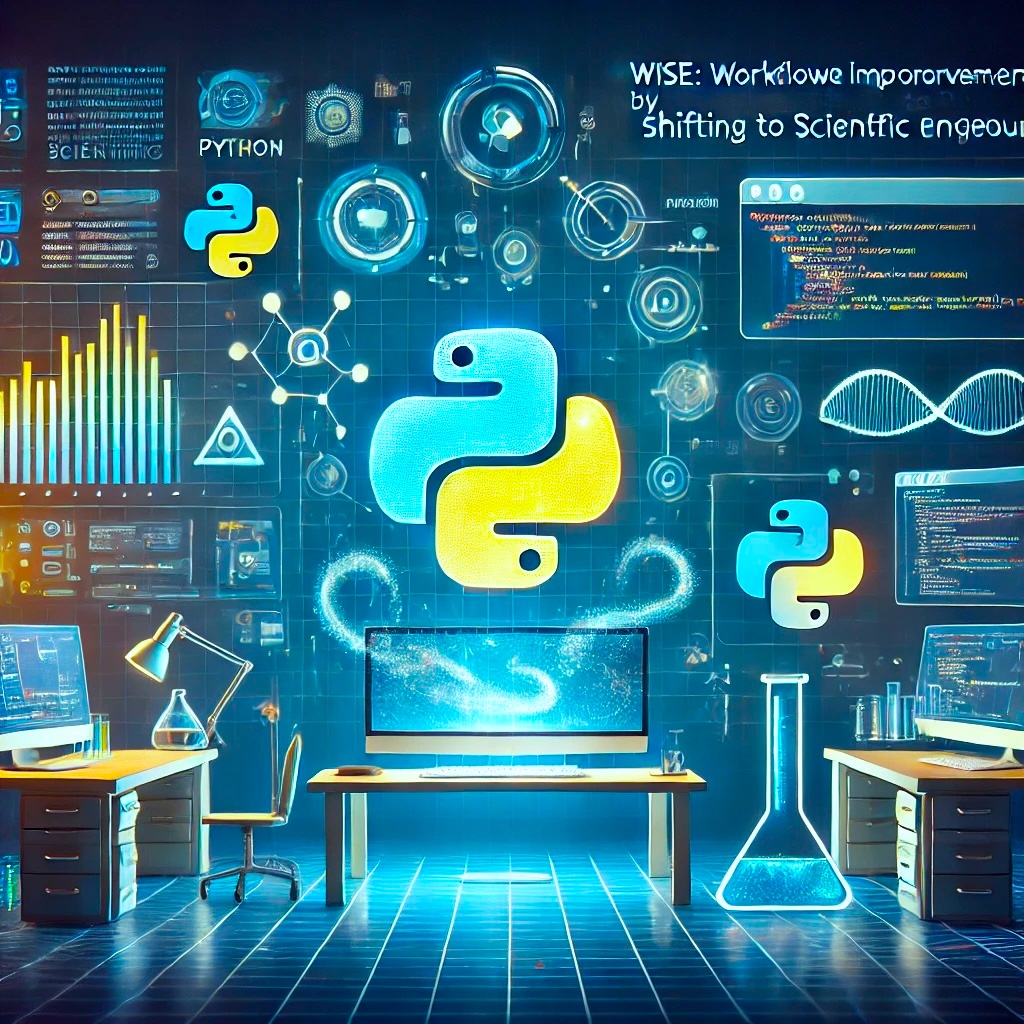
WISE: Workflow Improvement by Shifting to python in scientific Endeavors
by Alexandre Lainé & Cyprien Dautrevaux
Have you ever wanted, but lacked the time, to learn Python programming to update your data analyses and perhaps enhance them with new tools? In this era where open science is increasingly promoted, we are offering you the opportunity, over the course of two days, to delve into Python and its free tools. The idea behind this project is primarily collaborative; we aim to share our programming expertise to support you in what can sometimes be a long and challenging learning process. The objective is simple: by the end of these two days, we hope to provide you with a better understanding of Python’s role and value, help you begin transitioning some of your scripts and analysis methods, and offer suggestions to improve your workflows. For this project, no prerequisites or prior documentation are required. We will provide you with an interface and a ready-to-use environment so you can start programming, even from scratch. We look forward to seeing you motivated and eager to join this project. If you have any datasets you'd like to work on, feel free to bring them along, and we’ll be here to guide you. Get ready for coding!
Goals for the BrainHack:Getting use to Python and overall OpenScience, understand the different roles and interests of
Python programming in scientific studies, be proud of your own code.
-
A suitable and functioning Python environment (code environment, coding interface …)
-
Know where to find informations, and solve code issues
-
Know the good practices
https://mattermost.brainhack.org/brainhack/channels/bhg2024_wise-project
What will participants learn?Learn to use the Python language in the broad outlines, to be able to perform your own analyses and scripts (Statistics, Data exploration, Preprocessing, Automation, Machine learning, Regression …)
Data to useFeel free to bring your own datasets, and suggest your own analysis interest.
Number of collaboratorsmore
Credit to collaboratorsAll contributors are listed on the project's README.md file.
Typecoding_methods, pipeline_development
Development status0_concept_no_content
Topicother
ToolsJupyter, other
Programming languagePython
Modalitiesother
Git skills0_no_git_skills
Required skills
This is a project for beginners. No prior knowledge is required.: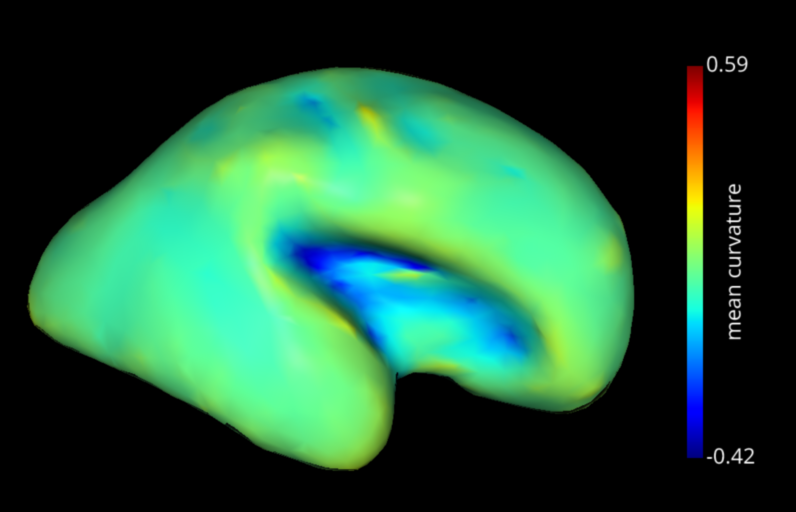
brain-SLAM
by Guillaume Auzias and the MeCA team
-
What are you doing, for whom, and why?
Our team develops the brain-SLAM python package designed for analyzing neuroimaging data using surface-base approaches. We improve it during hacking sessions and welcome external contributors! - What makes your project special and exciting?
The project is at an intermediate stage: not brand new but still not mature enough for spreading the world, let's move on! -
How to get started?
Send an email to guillaume.auzias-at-univ-amu.fr -
Where to find key resources?
https://brain-slam.github.io/slam/
https://github.com/brain-slam/slam
https://github.com/brain-slam/slam
Goals for the BrainHack:
- Update continuous integration tools using github actions
- Improve code testing and quality
- Propose new features
- Improve our coding skills
- Work collaboratively
- Have fun
-
any kind of unitest
-
any kind of tutorial
Will setup one on site
What will participants learn?
- Content and current status of brain-SLAM
- Collaborative work
Example data already included in the repos.
Number of collaborators3
Credit to collaboratorsAll contributors are listed on the project's README.md file. We will also discuss the writing of a paper.
Typemethod_development
Development status2_releases_existing
Topicother
Toolsother
Programming languagePython
ModalitiesMRI
Git skills2_branches_PRs
Required skills
This is a brainstorming and coding project, Python skills are required: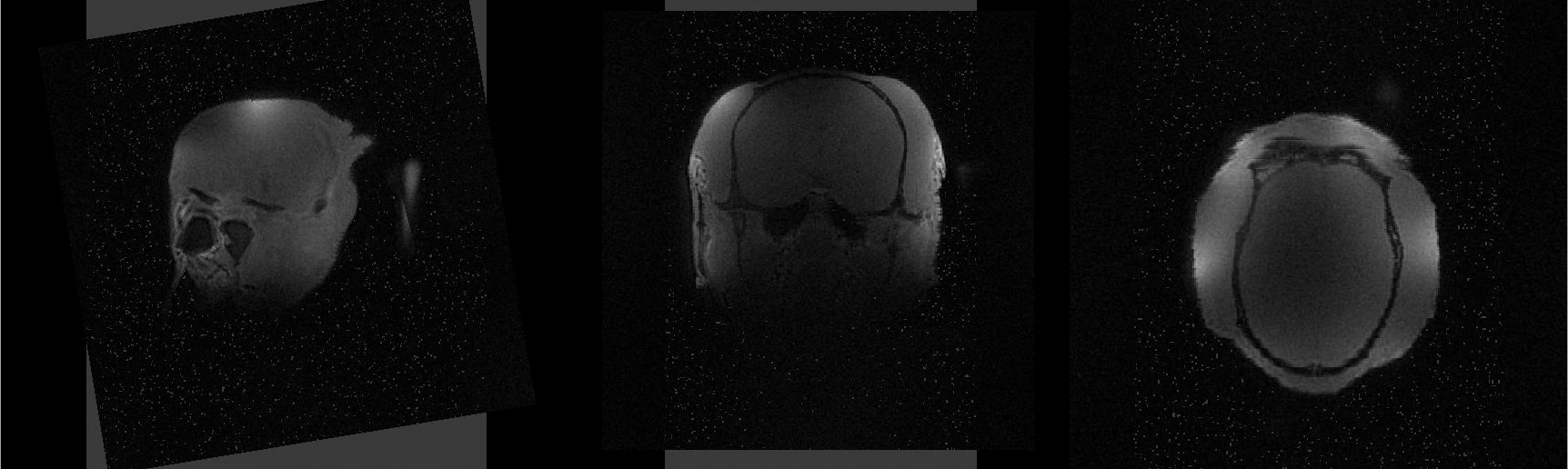
Bias correction of highly heterogeneous MRI images
by julfou81
davidmeunier79
Macapype is pipeline wrapping several software commands in order to segment non human Primate MR images. However in some cases, one of those command fails due to some extreme bias present in the images acquired with some high multidimensional array whose elements are small and in close proximity of the anatomy to be imaged. In some cases, when the vitamin pastille is close to the antenna, it is hyper intense and the usual commands for registering the images to the template fail.
Link to project repository/sources Goals for the BrainHack:We expect to robustify Macapype with respect to images with strong receive bias.
-
Visit this page for description of the origin of the problems encountered with those kind of acquisition:
https://github.com/PRIME-RE/prime-re.github.io/wiki/NHP-Issues
https://mattermost.brainhack.org/brainhack/channels/bhg24-marseille
Skills:
- bash
- FSL
- ANTs
- NiftyReg
- Python
When participating to this project, you will learn about MR images artifacts and how to correct them.
Data to useNHP data acquired from the CERMED can be used, also
Number of collaborators1
Credit to collaboratorsAll contributors are listed on the project's README.md file.
Typemethod_development, pipeline_development
Development status0_concept_no_content
TopicMR_methodologies
ToolsANTs, FSL, Nipype, other
Programming languageshell_scripting, unix_command_line
ModalitiesMRI
Git skills1_commit_push
Required skills
This is a brainstorming and coding project, Python skills are required.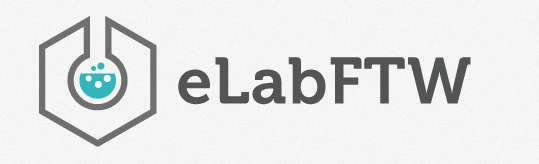
Electronic laboratory notebook presentation and discussion
Sylvain Takerkart & Laure Spieser & Christelle Zielinski & Sifaou fatai
Dipankar Bachar & Kevin Poirot
eLab is an electronic laboratory notebook for researchers. Which is useful for the acquisition of different experimental data/ metadata. Its aim is to no longer use a paper laboratory notebook and digitalize all the metadata related to experiments. This is useful for the data acquisition process by researchers. Also important in the data standardization process.
Link to project repository/sourceshttps://github.com/elabftw/elabftw
Goals for the BrainHack:The aim is the presentation of an electronic laboratory notebook: eLab. Allowing the acquisition of
experimental data/ metadata.
This presentation will be followed by a general discussion on the use of laboratory notebooks and
how to use
them.
https://mattermost.brainhack.org/brainhack/channels/bhg24-marseille-elabftw
What will participants learn?
- Electronic lab notebook
- Different ways to use it
more
Credit to collaboratorsEvery collaborator will have equal credits.
Typeother
Development status0_concept_no_content
Topicother
Toolsother
Programming languagenot_applicable
Modalitiesnot_applicable
Git skills0_no_git_skills
Required skills
This is a brainstorming project, non-coding skills are required.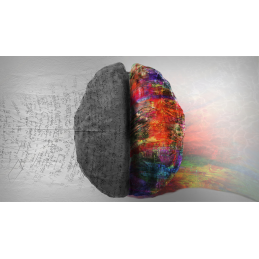
Beyond the Neurons: Exploring the Stars!
Rémi Bos
INPHIM facility
I aim to investigate calcium activity in two types of glial cells—radial glia (RG) and astrocytes—surrounding the central canal of the spinal cord. This region is known for its strong rhythmic activity, driven by neurons with high rhythmogenic capabilities, which arise from both intrinsic and network properties. These neurons are surrounded by astrocytes, which play a regulatory role in their rhythmogenic activity (Barbay et al., 2023). Additionally, research from Arulkandarajah et al. (2021) has shown that RG exhibit calcium spikes, unlike astrocytes. The key question is: How do radial glia and astrocytes interact during locomotion, and how do they influence pacemaker neurons in the spinal cord? This project seeks to uncover how glial cells contribute to the rhythmic dynamics of the spinal cord, offering insights into their roles in locomotion.
Link to project repository/sourceshttps://github.com/beyondNeurons/beyondNeurons
Goals for the BrainHack:To characterize the spatiotemporal dynamics of calcium signaling in these two glial cell types within the lumbar spinal cord.
- WP1: Segmentation of calcium imaging data to identify radial glia and astrocytes
- WP2: Analysis of the area under the curve (AUC) of calcium transients under three conditions:
- C1: Baseline (ACSF)
- C2: TTX treatment to block neuronal activity
- C3: TTX combined with a locomotor cocktail
- WP3: Mapping the spatial distribution (xy positions) of active astrocytes relative to the central canal
Communication channels:
https://mattermost.brainhack.org/brainhack/channels/bhg24-marseille-beyondNeurons
What will participants learn?
This project seeks to uncover how glial cells contribute to the rhythmic dynamics of the spinal cord, offering insights into their roles in locomotion.
The participants will learn (i) how to do segmentation of cell subtypes, (ii) exctraction and analysis of two-photon calcium imaging data, and (iii) locate active cells in a 2D field of view.
3
Credit to collaboratorsCo-authorship.
Typepipeline_development
Development status1_basic structure
Topicphysiology
Toolsother
Programming languagePython
Modalitiesother
Git skills4_not_applicable
Anything else?2P calcium imaging
Required skills
This is a brainstorming and coding project, Python skills are required: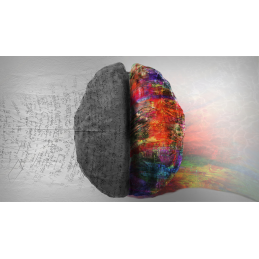
Understand and adapt the Hybrid Predictive Coding Model
Louis-Clément da Costa
Matthis Dallain
We aim to understand and adapt this model for timing studies, with a final goal to better understand delusions and halucinations in patients with Schizophrenia. I am currently a PhD student in the Contact (Control Timing and Action) Team at CRPN (Center for research in psychology and Neuroscience) under the supervision of Jennifer Coull working mainly on implicit Time perception.
Link to project repository/sources
The existing script is available here:
https://github.com/alec-tschantz/pybrid
To characterize the spatiotemporal dynamics of calcium signaling in these two glial cell types within the lumbar spinal cord.
- Understand in deep how the model is working
- Adapt the model for a sound dataset
- Disrupt the model's predictions
Communication channels:
https://mattermost.brainhack.org/brainhack/channels/hpc-hybrid-predictive-coding-model
What will participants learn?I am a beginner in Python, so we will be at the same level or you will probably be much better than me at coding. We will learn together how this model is build through coding and discussing.
Number of collaborators4
Credit to collaboratorsProject contributors will be listed in the readme file and thanks in my PhD manuscript
Typeother
Development status0_concept_no_content
Topicbayesian_approaches, deep_learning
ToolsJupyter
Programming languagePython
Modalitiesother
Git skills0_no_git_skills
Required skills
This is a brainstorming and coding project, Python skills are required:Team
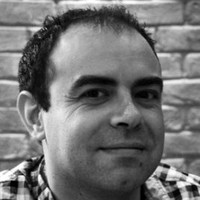
David Meunier
Research Engineer
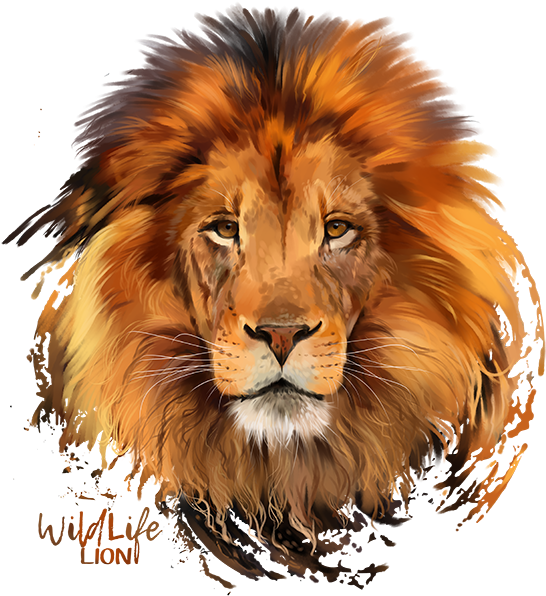
Dipankar Bachar
Research Engineer
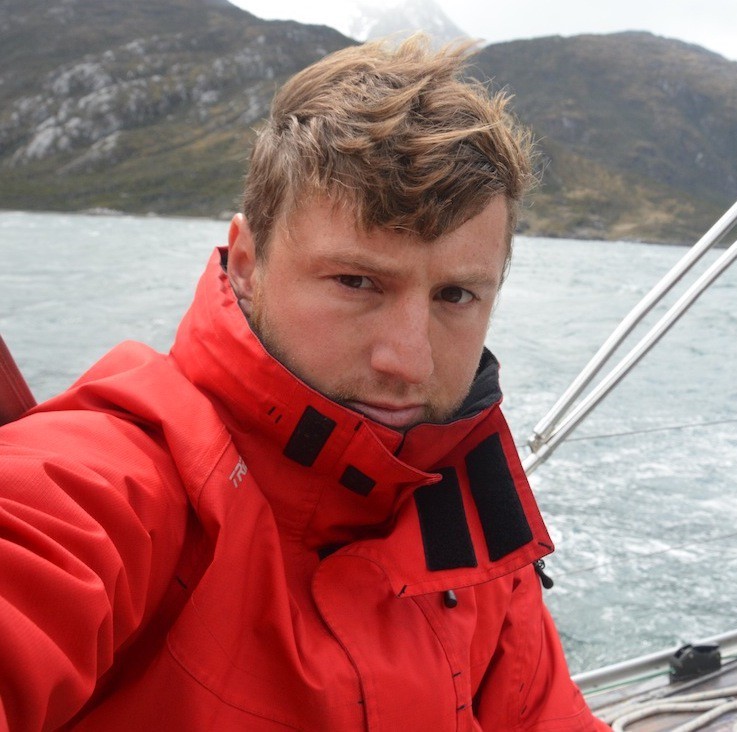
Matthieu Gilson
Junior Professor
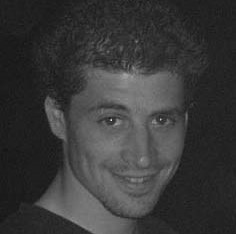
Manuel Mercier
Research Associate
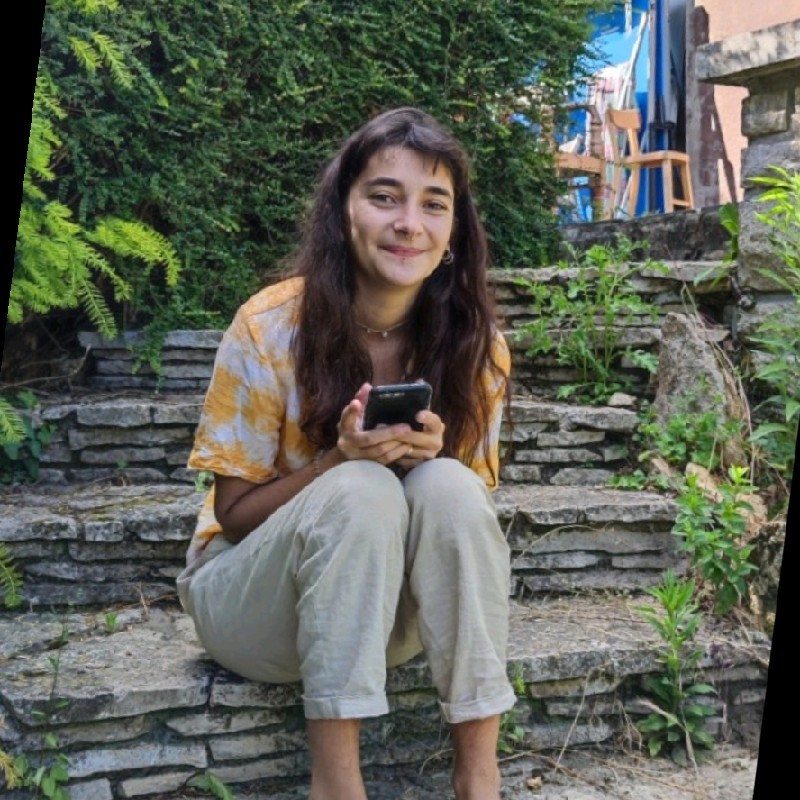
Laurie Mifsud
PhD student
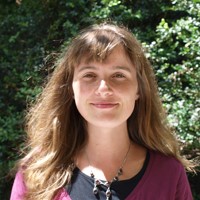
Christelle Zielinski
Data Analysis Engineer
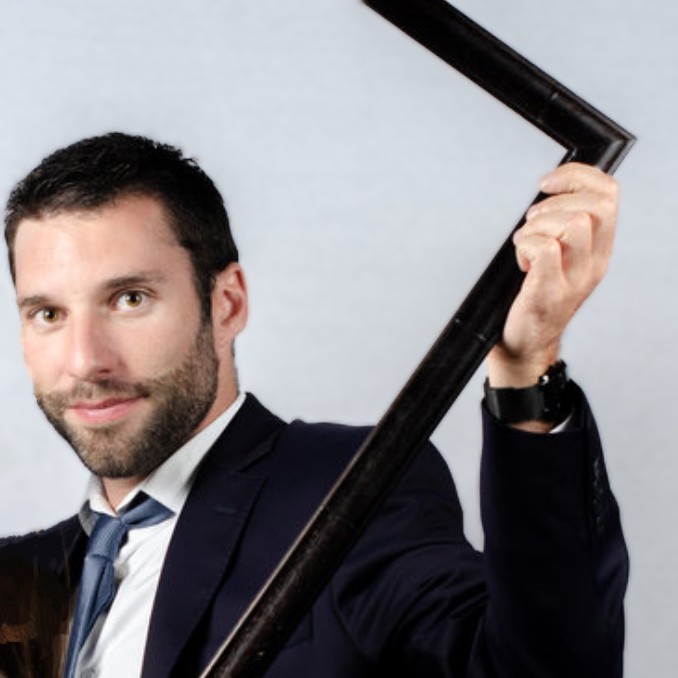
Hugo Dary
Research Engineer
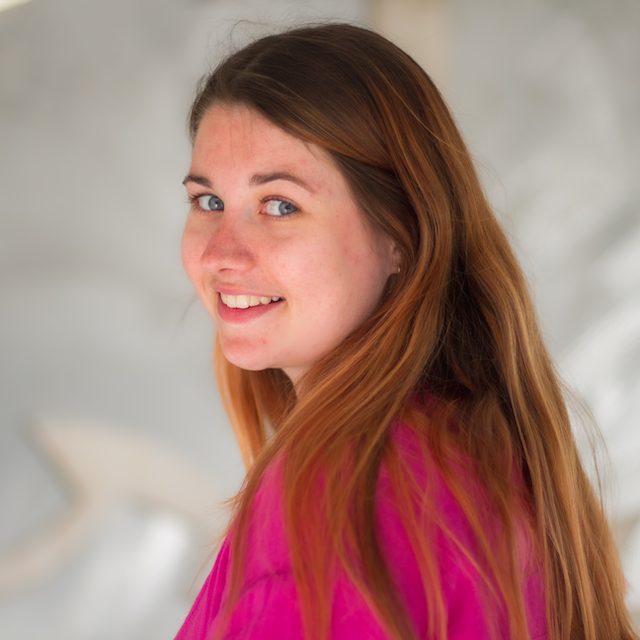
Marie Bourzeix
PhD student
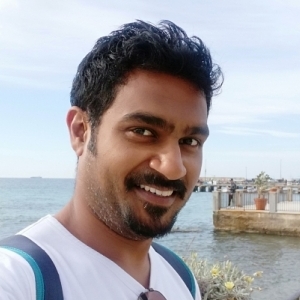
Shailesh Appukuttan
Postdoc
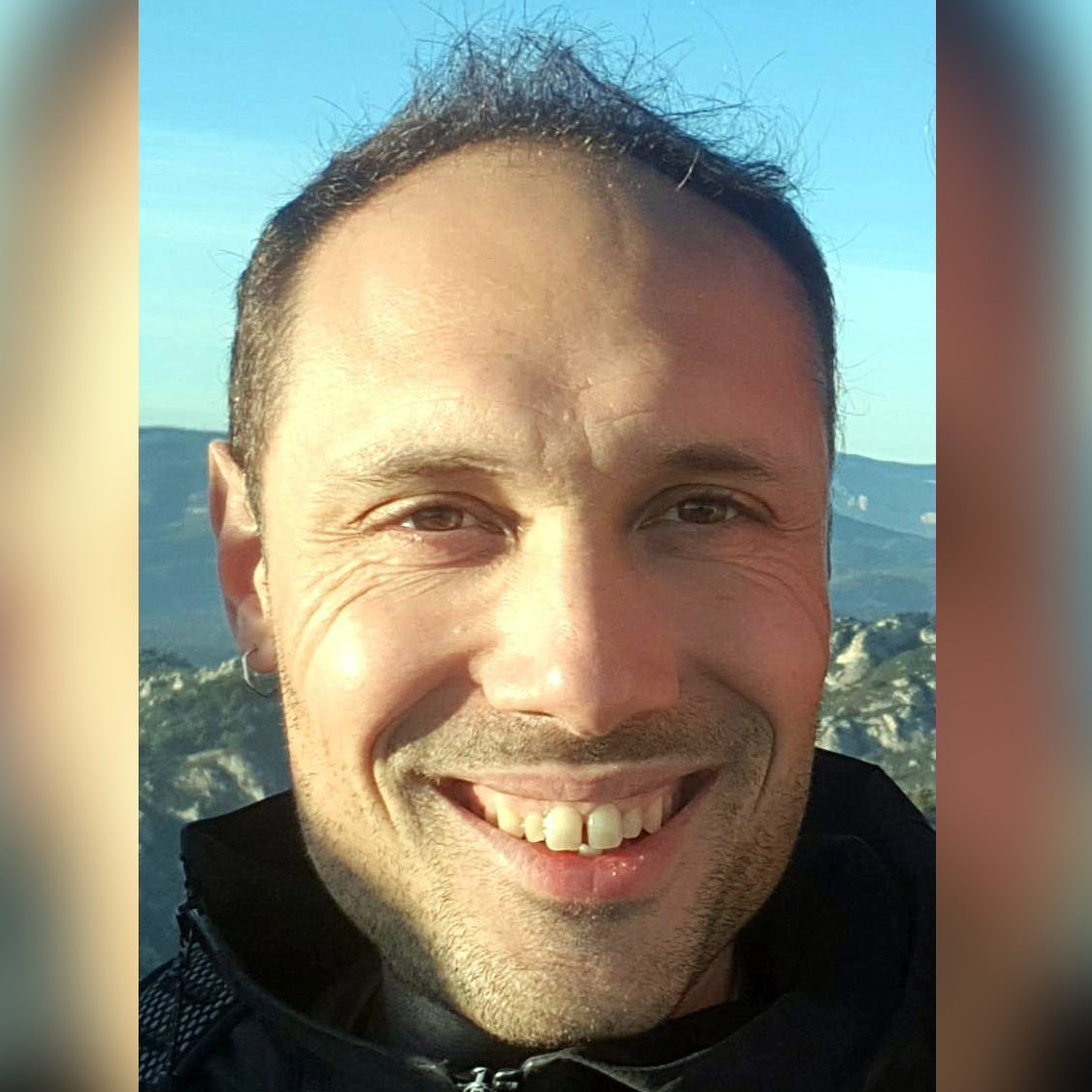
Giorgio Marinato
Postdoc
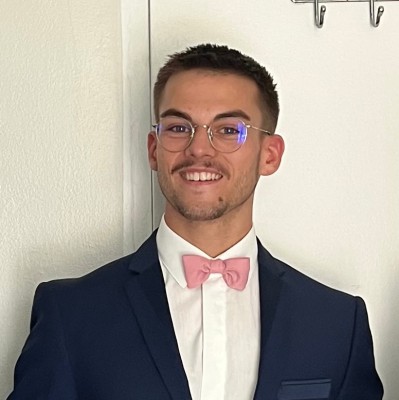
Cyprien Dautrevaux
PhD student
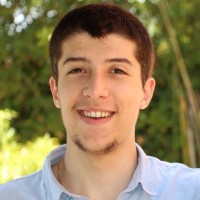
Alexandre Lainé
PhD student